Demo - Bayesian Network Simulation
Bayesian Network Simulation
Overview
This simulation visualizes a Bayesian Network, a probabilistic graphical model used for decision-making under uncertainty. It consists of nodes (random variables) and edges (dependencies between variables), forming a directed acyclic graph (DAG).
How It Works
Each node represents an event, such as Cloudy Weather, Rain, or Traffic Jam.
The edges define causal relationships, e.g., Rain increases the chance of Grass being Wet.
The simulation calculates probabilities of different events occurring, updating their values dynamically.
Node colors change based on their probabilities: lighter shades = lower probability, darker shades = higher probability.
The graph rearranges itself automatically using different layouts, showing how structures adapt dynamically.
Applications
✔ Machine Learning & AI → Used in predictive modeling and reasoning under uncertainty, such as fraud detection and disease diagnosis.
✔ Risk Assessment → Helps industries like finance, insurance, and cybersecurity predict possible outcomes.
✔ Medical Diagnosis → Doctors use Bayesian networks to evaluate symptoms and diseases, improving diagnostic accuracy.
✔ Autonomous Systems → Supports fault detection & predictive maintenance by assessing system health under uncertain conditions.
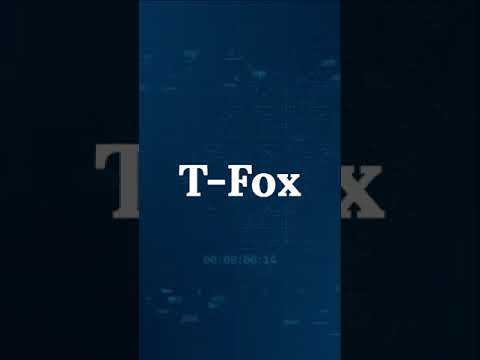
Consulting
Research and development in physical, engineering, life sciences
© 2024. All rights reserved.
San Jose, CA
US